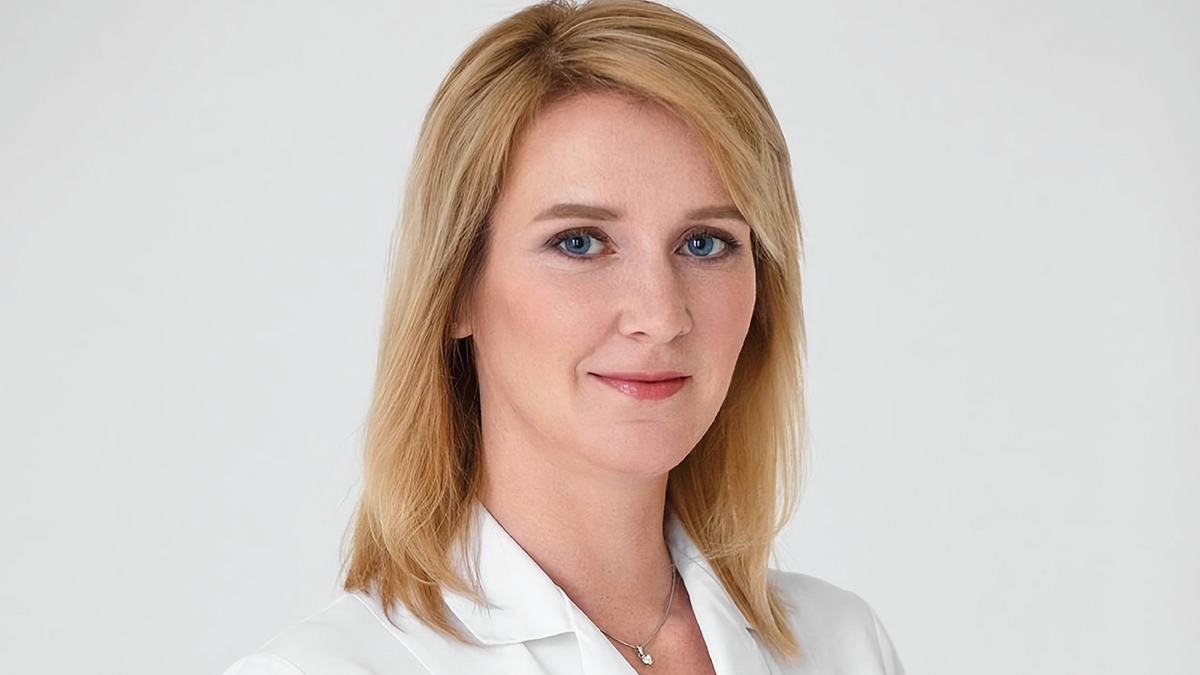
Advanced technologies have become a routine part of our everyday life – just as they will drive the transformation of the eye care industry. Digital transformation in eye care already affects diagnostics, clinical research, treatment, consultation, communication, and more. In this article, I’d like to focus on the automation of retina scan analysis (fundus and OCT) with the help of artificial intelligence (AI).
AI-powered automation of fundus photo and OCT scan analysis is used by several companies, including Eyenuk, Optina Diagnostics, IDx Technologies, and Visulytix, to diagnose eye diseases, such as diabetic retinopathy, macular degeneration, and glaucoma. In short, these companies use machine learning algorithms to analyze retinal images and detect abnormalities. And their technology can potentially improve patient outcomes by identifying eye diseases earlier and more accurately.
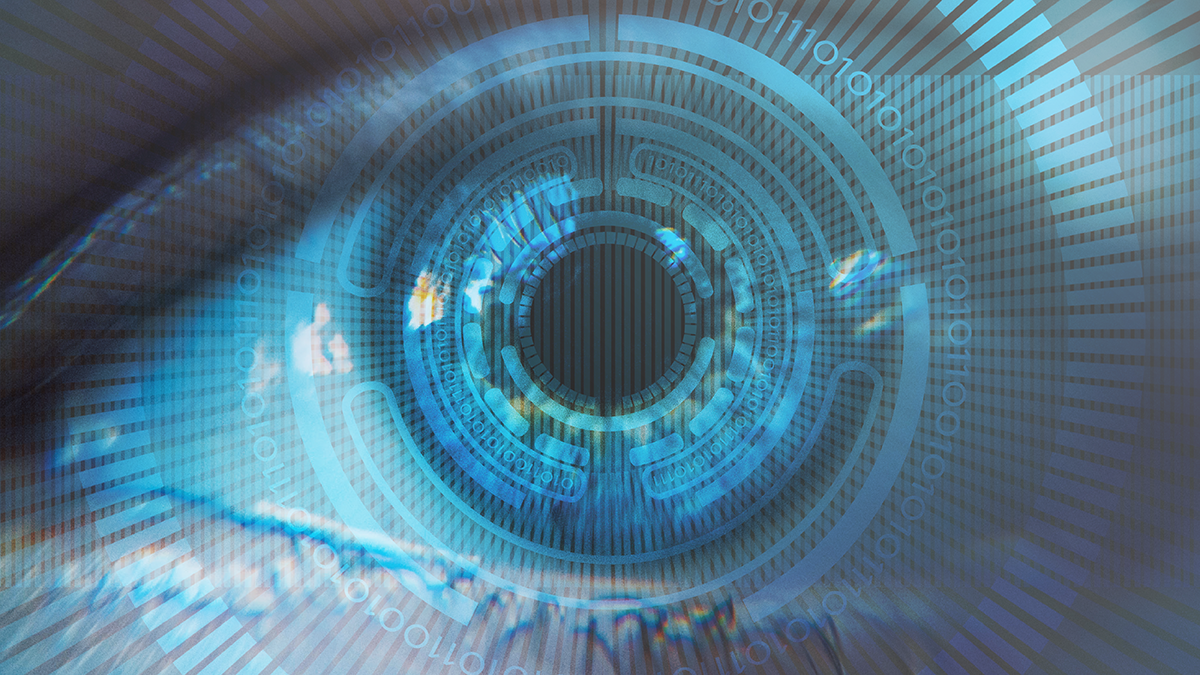
OCT is considered the most accurate method of retina scan analysis because of its ability to provide such detailed images. OCT can detect even the most subtle changes in retinal thickness and structure – changes that can signify early retinal disease. And earlier detection can lead to more effective treatment and better outcomes for patients.
Though OCT is “the best,” it is also the most complicated method of retina scan analysis – it takes months, if not years to fully master it. And that’s why automated OCT scan analysis is so interesting. To create a platform that can detect over 100+ retina pathologies and pathological signs, Altris AI collected thousands of OCT scans from my eye clinic in Kyiv, Ukraine, and from other partner eye clinics. A team of retina experts began labeling OCT scans, marking pathologies and pathological signs. On the basis of this labeled data, the company’s AI algorithm was taught to find these pathologies. It took five years for Altris AI to complete the process, reaching 91 percent accuracy in OCT scan analysis.
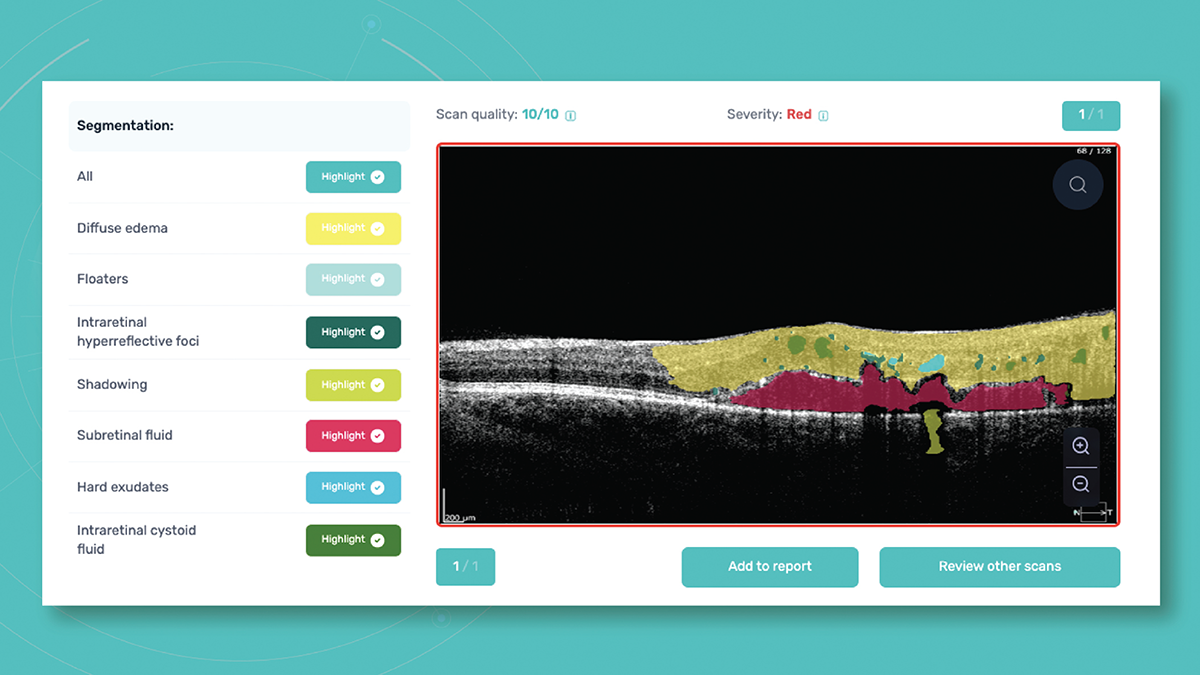
Where does AI-enabled automated analysis fit into the clinical picture?
- Better patient triage. AI algorithms can help triage patients by analyzing OCT images and identifying those who require urgent attention. For example, AI can identify signs of macular edema – a serious condition requiring immediate treatment.
- Automatic segmentation. AI algorithms can automatically segment OCT images, which can help to improve the accuracy and consistency of the analysis. This can save time for clinicians and improve the overall quality of care.
- Measurement of lesions. AI algorithms can measure lesions in OCT images, which can help clinicians to monitor disease progression and evaluate the effectiveness of treatments. For example, AI can measure the size and extent of drusen in patients with age-related macular degeneration.
- Recognition of biomarkers and patterns. AI algorithms can identify biomarkers and patterns in OCT images that may be difficult for clinicians to detect. For example, AI can identify patterns of fluid accumulation in patients with diabetic macular edema.
There is a final point to make: AI will never be a substitute for living, breathing eye care professionals. Only optometrists and ophthalmologists can combine the necessary diagnostic approach with real-world experience, communication skills, and empathy. AI is a tool, like a tomograph or a slit lamp, that can provide more information and improve patient outcomes, but the final diagnosis must always be the responsibility of a specialist.